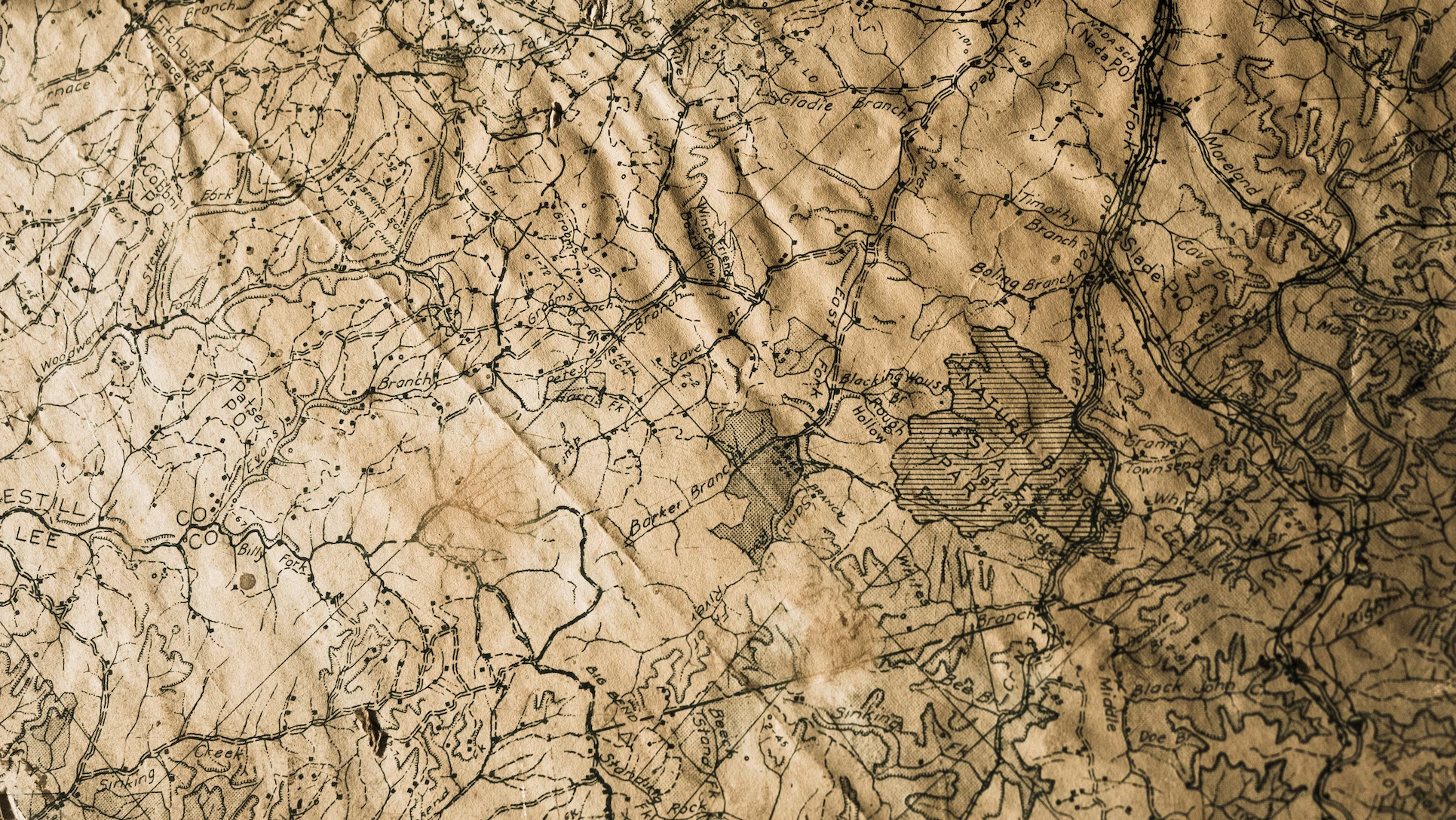
Description
The initiative delves into evaluating the efficiency and efficacy of various mapping methods—optimal interpolation, machine learning techniques, and data assimilation—for the purpose of reconstructing simulated observations. The observations in question are sourced from several realistic numerical models like CMEMS reanalysis, WMOP, and eNATL60. The end goal is to generate 3D gridded fields that provide a comprehensive spatial understanding of the studied marine phenomena.
Impact During the Project
Richer Data Reconstruction:
Traditional State: Historically, a singular or limited set of methodologies might have been employed for mapping and reconstructing observations, potentially yielding limited or biased reconstructions.
Advancement: By comparatively studying diverse mapping methodologies, this initiative ensures that the most effective technique is employed for specific types of data, thereby enhancing the richness and accuracy of reconstructed data.
Utilizing Modern Computational Techniques:
Traditional State: Traditional mapping techniques like optimal interpolation have been the norm for many marine observational reconstructions.
Advancement: By introducing and comparing machine learning techniques and data assimilation, the initiative taps into the potential of advanced computational methodologies, leveraging their capability to handle vast datasets and intricate patterns.
Impact Post Project
Improved Model Validity:
Traditional State: The validity and accuracy of marine models like CMEMS, WMOP, and eNATL60 heavily depend on the techniques employed for data reconstruction.
Advancement: Ensuring that the best mapping method is used based on the nature of the data and the model can significantly enhance the validity and accuracy of these marine models, leading to more reliable marine insights.
Bridging Computational Advances with Oceanography:
Traditional State: There has often been a lag in incorporating the latest computational techniques into marine science disciplines.
Advancement: By integrating machine learning techniques with traditional mapping methodologies, this initiative bridges modern computational science with oceanography, ensuring that marine studies remain at the forefront of technological advancements.
Advancement over and above State of the Art
The comparative exploration of various mapping techniques for reconstructing simulated observations heralds a more nuanced approach to marine data analysis. Instead of relying solely on traditional methods, the initiative recognizes the potential of modern computational techniques and seeks to integrate them effectively.
This doesn’t just improve the quality of reconstructed data but also broadens the scope of insights that can be derived from it.
In essence, it signifies a fusion of time-tested methodologies with the latest in computational science to achieve a richer, more detailed, and more accurate understanding of marine systems.
Links and References
NA